CNH Monthly Roundtable Talk (Mar) by Dr Sophie Lin & Haomin Chen
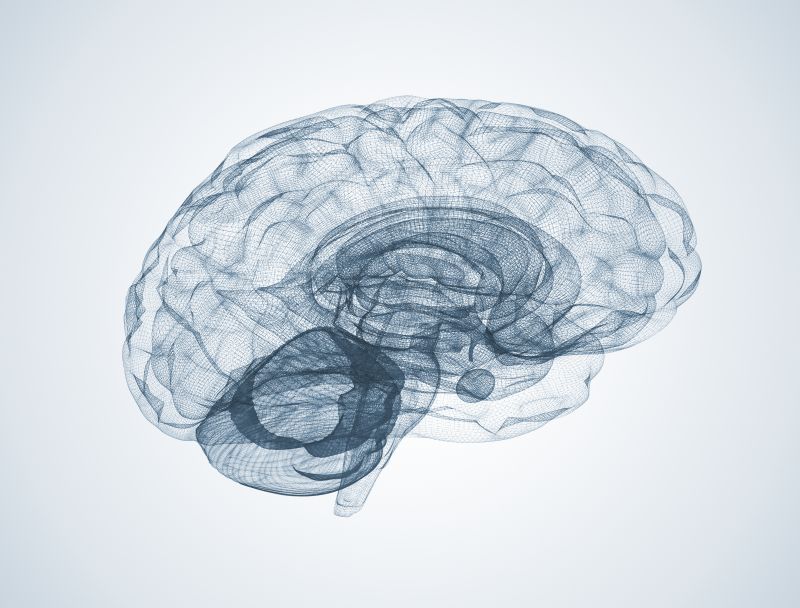
Lecture capture is now available
Haomin Chen graduated from the University of Melbourne with a B.A. majoring in Psychology in 2019, and obtained a 1st Class Honours in Psychology in 2020. Haomin is currently a 3rd year PhD student working under the supervision of Dr. Adam Osth in the Melbourne School of Psychological Sciences at the University of Melbourne. Her research is focused on investigating the three-way relationship between confidence, response latency and accuracy using a decision model.
Linear ballistic accumulator models of confidence and response time
Accurate decisions tend to be both confident and fast. Nonetheless, there are relatively few models that can simultaneously address this three-way relationship, especially for single stage decisions where participants indicate both their choice and their confidence. Extending on a common decision architecture of the linear ballistic accumulator framework, two models have been proposed – 1) a Multiple Threshold Race model which instantiates the Balance-of-Evidence hypothesis where confidence is determined through the difference between accumulated evidence for competing options (e.g., Reynolds, Osth, Kvam, & Heathcote, in revision), and 2) a newly developed Confidence Accumulator model which assumes that confidence itself is accumulated independently for each confidence option. To test these two confidence architectures, we ran two experiments manipulating the length of the confidence rating scale across 2-, 4-, or 6-options in a recognition memory task along with a perceptual task. Different models were compared that made different allowance for how the length of the confidence scale affected model parameters. While both model classes found that thresholds were affected by the length of the scale, drift rates were only minimally affected. Implications for models of confidence and response time will be discussed.
Dr. Sophie Lin is interested in how the brain identifies structures, acts and adapts, through the Predictive Coding framework, in a world with uncertainty. She uses perceptual decision tasks, computational modelling and functional neuroimaging to study these questions. Outside the Lab, she sometimes conduct real-life uncertainty ‘fieldwork’ at a local kickboxing club.
Validation of Bayesian strategy in probabilistic inference by evaluating the ability to generalise knowledge
Numerous studies have found that the Bayesian framework, which formulates the optimal integration of the knowledge of the world (i.e. prior) and current sensory evidence (i.e. likelihood), captures human behaviours sufficiently well. However, there are debates regarding whether humans use precise but cognitively demanding Bayesian computations for behaviours. Across two studies, we trained participants to estimate hidden locations of a target drawn from priors with different levels of uncertainty. In each trial, scattered dots provided noisy likelihood information about the target location. Participants showed that they learned the priors and combined prior and likelihood information to infer target locations in a Bayes-fashion. We then introduced a transfer condition presenting a trained prior and a likelihood that have never been put together during training. How well participants integrate this novel likelihood with their learned prior is an indicator of whether participants perform Bayesian computations. In one study, participants experienced the newly introduced likelihood, which was paired with a different prior, during training. Participants changed likelihood weighting following expected directions although the degrees of change were significantly lower than Bayes-optimal predictions. In another group, the novel likelihoods were never used during training. We found people integrated a new likelihood within (interpolation) better than the one outside (extrapolation) the range of their previous learning experience and were quantitatively Bayes-suboptimal. We replicated the findings of both studies in a validation dataset. Our results showed that Bayesian behaviours may not always be achieved by a full Bayesian computation. Future studies can apply our approach in different tasks to enhance the understanding of decision-making mechanisms.